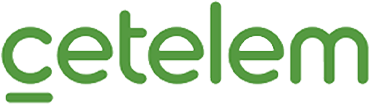
Cetelem: how to get to the right persona by
combining machine learning and 1st party data?
Cetelem: how to get to the right persona by combining machine learning and 1st party data?
Cetelem: how to get to the right persona by combining machine learning and 1st party data?
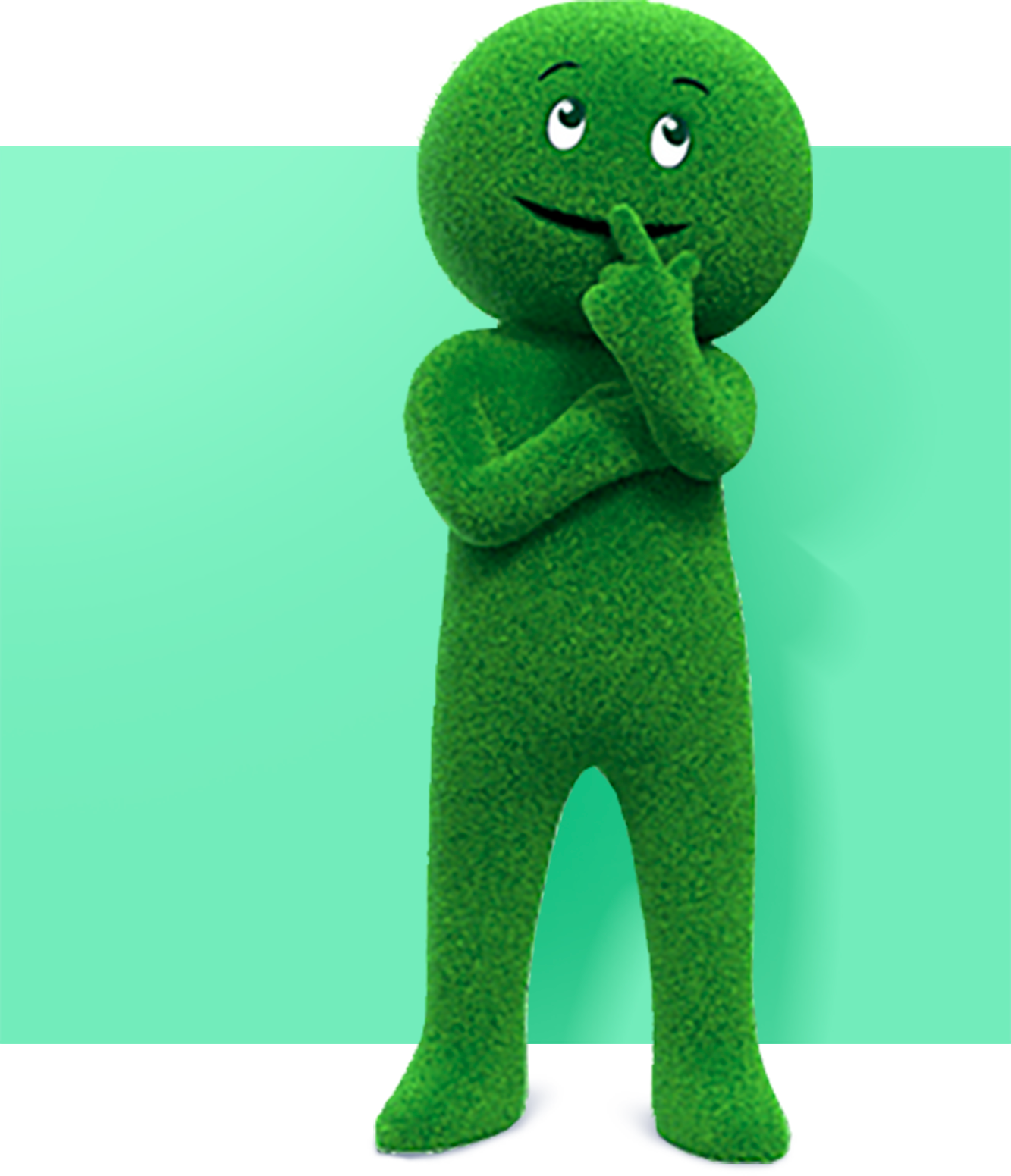
This advertiser is active in the financial sector, with their core business being all types of credit loans (car, renovation, etc).
The specificity of this industry is related to the challenges they’re facing regarding strict GDPR regulations as well as technical difficulties inherited from a historic way of work.
The technical difficulties are strongly linked to closing the loop between an online credit request (a lead) and the actual contract (which is signed in a classical, “offline” way).
The conversion funnel is thus split over
both online and offline channels
The conversion funnel is thus split over both online and offline channels
A potential customer would first have to submit a credit request online. Once that’s submitted, there is a first layer of qualification: if this person has a history of debts with the Belgian National Bank or if they show other signals of being a risk for giving out a loan, they will automatically be refused. If they pass this first automatic filtering, they are allowed to actually request a credit loan online, which is sent via postal mail for signature. The rest of the process happens offline, meaning there is no way of identifying the transformation rate from requesting a credit and actually signing the contract and becoming a client.
For this client the business objective is to find new customers that are qualified in terms of reimbursement capabilities. Over the years, we noticed a steady increase of incoming visitors applying for a credit request (which was the primary conversion) but not necessarily passing through the first acceptance filter. This means that machine learning is of extreme importance in order to identify the most compatible audiences on SEA. In other words, a focus needs to be put on the potential clients who will already pass through this first automated acceptance process. In order to face this challenge and focus on quality of leads rather than on a quantity of users entering the conversion funnel, we needed to adapt the way the bid strategies were set up in Google Ads.
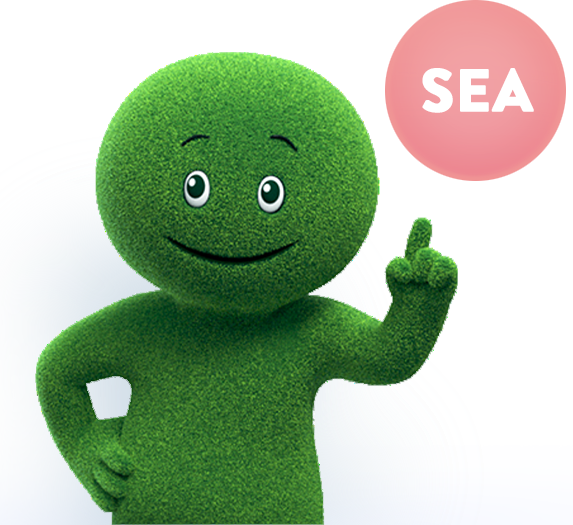
Therefore we registered two
conversions as the main goal
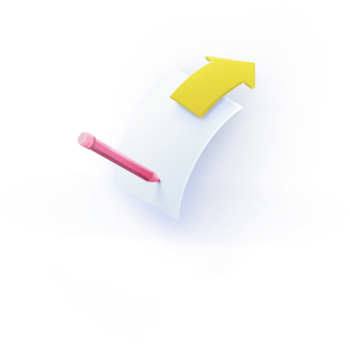
People submitting the credit
request form
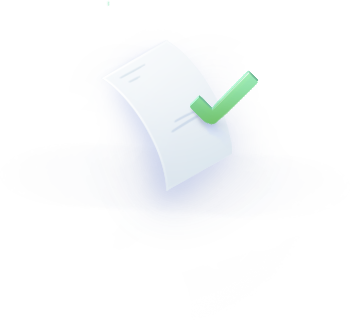
People being accepted automatically
for said credit request
This resulted in the algorithm being fed with much more information then
before to really understand both groups and find out what differentiated
them. That way, the most valuable clients were more easily identified and
captured by Google Ads and it showed in the results:
This resulted in the algorithm being fed with much more information then before to really understand both groups and find out what differentiated them. That way, the most valuable clients were more easily identified and captured by Google Ads and it showed in the results:
This resulted in the algorithm being fed with much more information then before to really understand both groups and find out what differentiated them. That way, the most valuable clients were more easily identified and captured by Google Ads and it showed in the results:
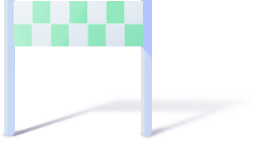
The transformation rate (from sending a credit request and being accepted) increased by a whopping 35%.
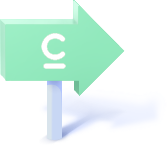
The conversion volume of accepted leads increased by 40%.
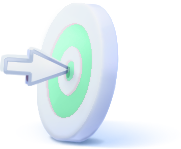
And led to a CPA which was 37% lower than before the change was made in the bidding strategy.
This way of working where we pushed the bidding strategy in a certain direction really showed off the results.
Now the next step would be to go one step further and actually close the loop with the final contract being signed offline, by importing this data automatically into Google Ads. Once the algorithm has all the data for this type of audience, the targeting could become even more precise by optimizing on the actual contracts rather than the accepted credit requests, inevitably resulting in an increased ROI for this client.
The first steps have already been made towards the quest of feeding the algorithm with more specific data; starting with the allocation of a unique ID for each and every credit request that happens online and keeping this ID towards the entire approval and signature process, allowing to connect the dots between online leads and offline contracts.